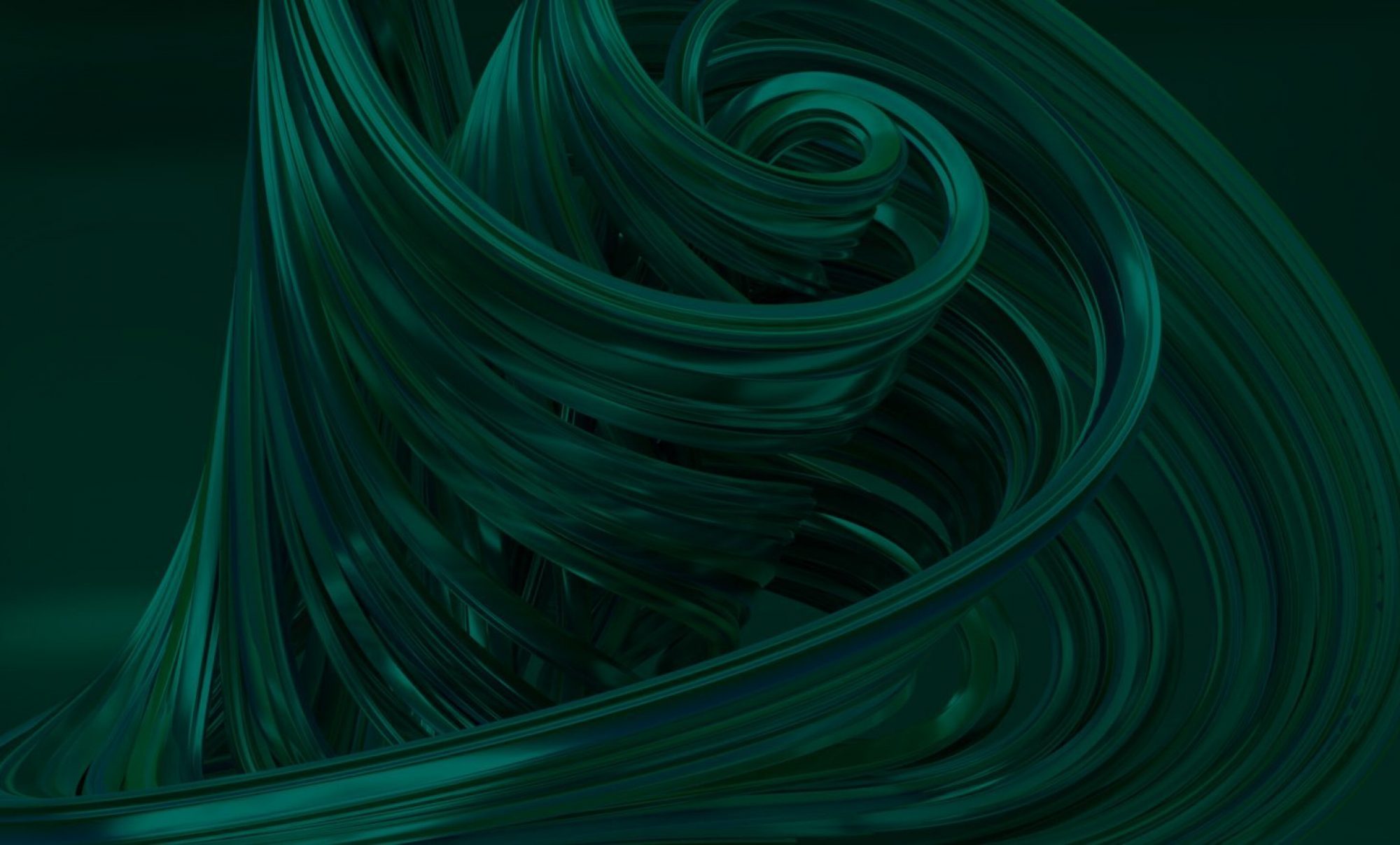
How data science initiatives get killed (and why they still need to be done)
Current advances in data science open up a lot of new opportunities. Companies learn to leverage their data to improve business processes and products. It changes the competitive landscape. So everyone with an innovative mindset is looking for ways to cash in on this technology. But all too often, it turns out that short term interests can block the road to the benefits of data science. I came across some interesting cases recently that illustrate what sort of issues may arise.
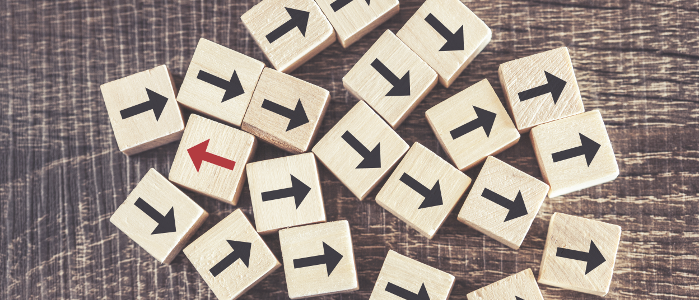
“Data science? Not our task”
I recently met an asset manager on a conference. She is responsible for the buildings and infrastructure of a large institution. She intends to improve the overall efficiency of the asset management with analytics. She wants to collect all asset data in a central data store and then analyze it to find efficiency gains.
However, her company has outsourced the operational side of the asset management to various suppliers. These contractors are wary of sharing information because it would give the asset managers uncomfortably detailed insight in their performance.
Also, the colleagues of this asset manager are not interested. They state that their job is to optimize asset management by negotiating the best possible agreement with contractors. The idea is that the contractors will optimize their own work to make the best possible offer. The asset managers should not bother with the way in which the contractors do their work.
Would a central repository indeed conflict with the outsourcing principle? I think not: in the larger picture both the suppliers and the contract managers have a joint interest in the best possible asset management.
Suppliers who do not want to maximize their efficiency will sooner or later be pushed out by more efficient competitors. Contract managers who do not want to learn about the process as a whole will never be able to exploit synergies. Their asset management will always be more expensive than necessary.
“All profit should be mine”
I met a manager in a company that builds machines. He told me that he wants to move from supplying machines to providing the service that their machines deliver: “servitization”. The idea is that a user is not so much interested in having the machine but rather in what the machine does: the service it provides. Users are willing to pay for a guaranteed service level. Rather than just selling the machine, the supplier can also sell the guaranteed service level and then use big data analytics to organize his own processes such that this service level is attained at minimum costs.
At one point this manager offered the machines as a service to one of his clients. It was an attractive offer: altogether the client would be paying just 50% of the costs that he was now paying annually for the purchase of the machines. Surprisingly, the client turned him down flatly.
The reason was that the client thought that the supplier would benefit too much from the service-based contract. Part of the deal was that the contract would be in place for a relatively long period. That would give the supplier the time to benefit from data science by reaping the benefits of predictive maintenance. But the client argued that this would probably be successful anyway and that he would be paying too much in the long run.
Like in the previous case, there is no doubt that data science can benefit all. The question here is who will benefit most. In this case, the supplier will probably be obliged to innovate with data science anyway. If not, his competitors will likely overtake him. Therefore, the client made the logical assumption that it’s better to negotiate in a few years’ time when the impact of new technology is clear.
However, by playing the game too hard, the client might also make it difficult for the supplier to finance this innovation. It would be useful if the client also saw the bigger picture here.
“Not too much insight, please”
My last example is one that I heard during a talk on the IoT tech day, last year in Utrecht. The speaker represents a group of volunteers that develops inexpensive devices to measure air quality.
The idea is that these devices will be so affordable that many people will want to have them to check the air quality around the house. Gathering the data from all these devices would give the city (in this case Ghent in Belgium) a detailed real-time map of the city’s air quality. With this information, the city could initiate projects to improve the situation. Isn’t that a wonderful application in the field of smart cities?
Alas, this initiative is not popular with everyone. There is fierce resistance from home owners in strongly polluted areas. This is exactly the group of people that could benefit most. However, they fear that the value of their houses will decrease when potential buyers could see how bad the air quality is. Clearly, these residents are not convinced that the city will act on the data effectively. Therefore, they prefer that the air quality in their neighborhood remains unknown.
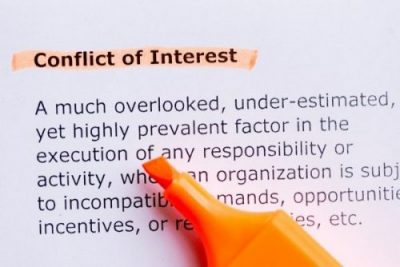
A balance of interests, as always
These three examples show that introducing data science is not always trivial, even if there are clear benefits. The objections can be valid in the short term. The asset management suppliers might expose their inefficiencies. The client of the machine supplier might pay too much for innovation that will happen anyway. The home-owners might lose value on their real estate.
However, these objections are less valid when thinking about the broad picture. The asset management suppliers would learn about their inefficiencies and become more competitive. The client of the machine builder would stimulate innovation and possibly get bigger rewards in the future. The homeowners could use data science to force the city council to improve air quality.
The trick probably to arrange the proper contracts that will balance the short term disadvantages with long term benefits.
In the end, all parties involved are learning how to deal with data science, its benefits and its pitfalls. At VORtech, we help companies in this learning process. That’s part of what makes our work so interesting: the potential is huge but daily practice is always a challenge.
Mark Roest
Links
- Blog: Big Data MBA.
- Blog: Data science for utilities: opportunities and challenges.
- Page: Data Science.
- Page: Software services.
- Page: Consultancy.