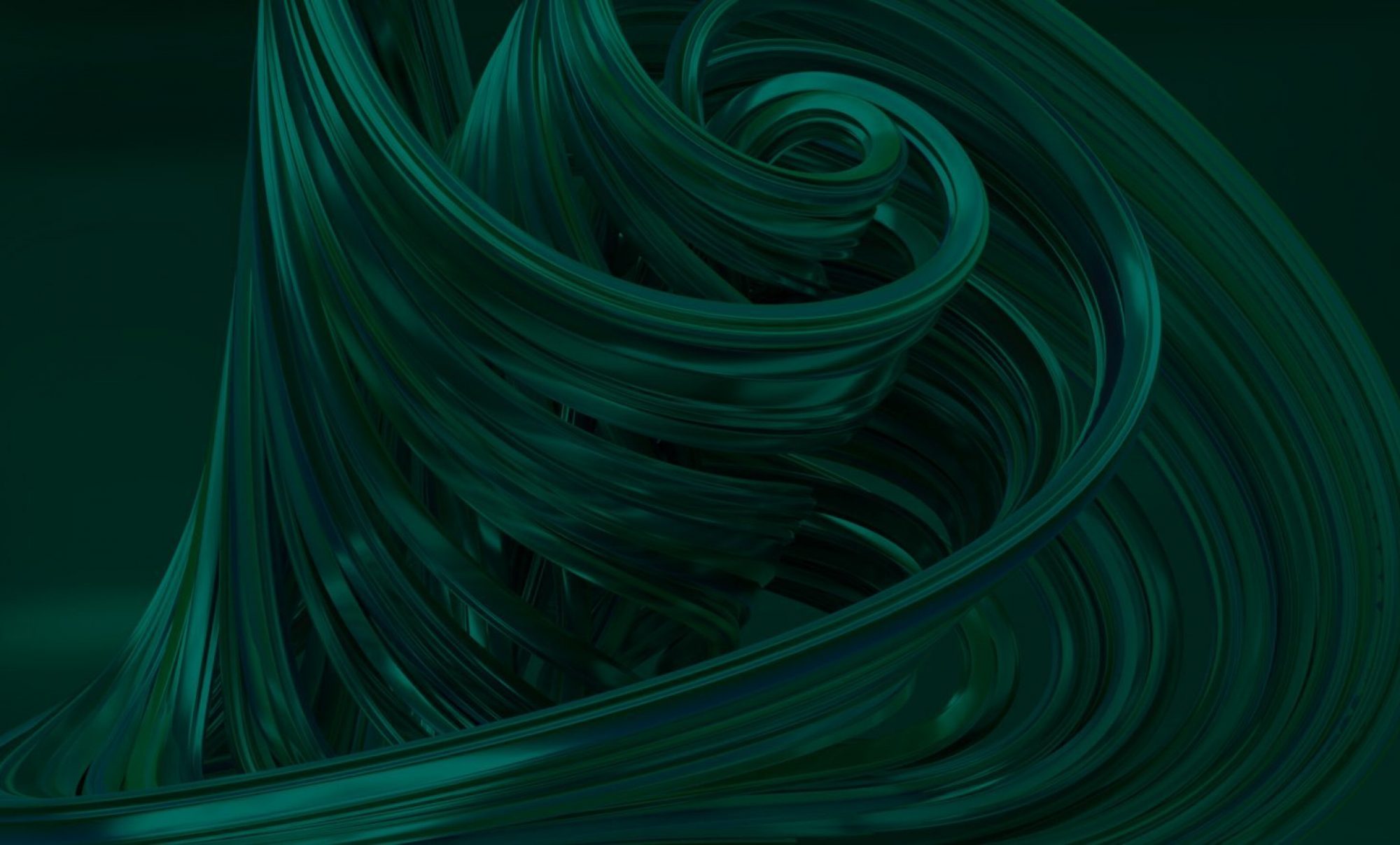
Projects
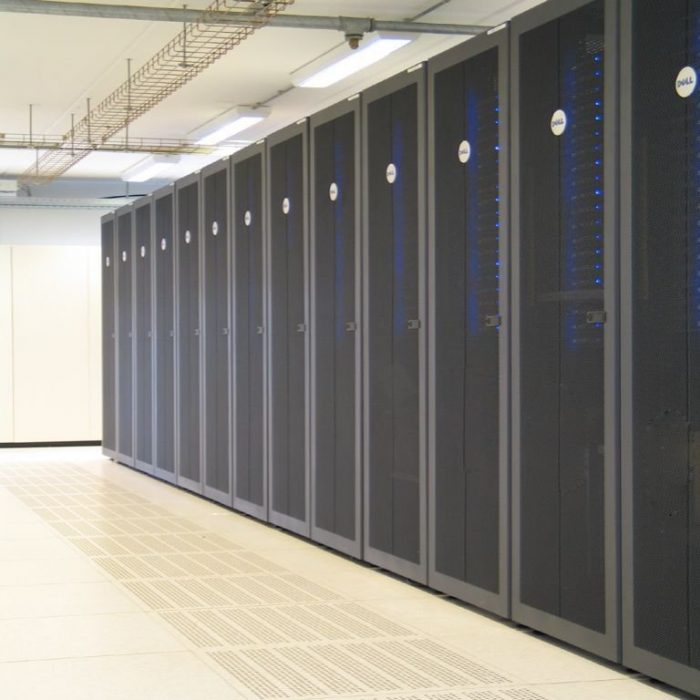
A digital twin for the indoor climate in data centers
4DCOOL is a digital twin for the indoor climate in the server rooms of data centers. It provides an actual and accurate 3D view of the temperature and flow. In addition 4DCOOL allows operators to run what-if scenarios. This lets them play with settings for the cooling so as to minimize costs and avoid local hot spots.
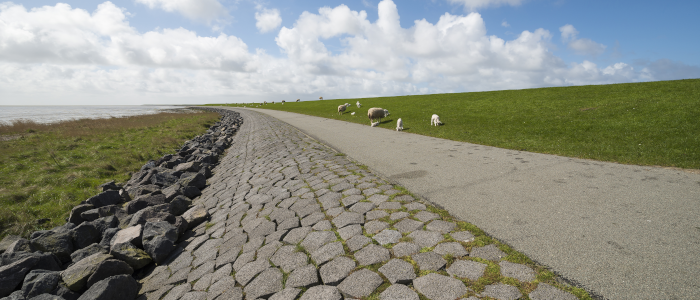
Speedup of software for assessing flood defenses
Every 12 years, the Dutch Department of Water Ways and Public Works assesses the strenght of the flood defenses, using the Hydra Ring software. VORtech realised a significant speedup of the software, using both code optimisations and algorithmic improvements.
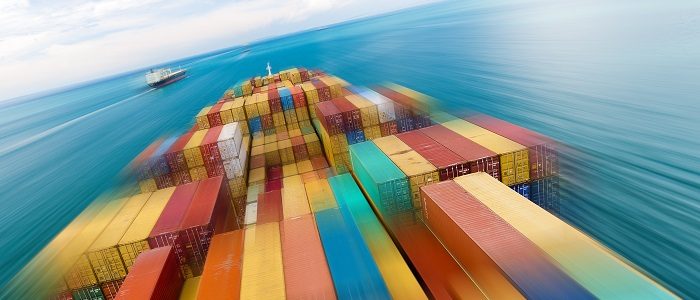
Speedup of software for ship design
There is continuous pressure on the time available to design a new ship. But at the same time, the requirements on the designs are increasing. Being a knowledge institute, Marin is constantly working on reduction of the time needed for a design. VORtech helped to speedup one of their most important design codes.
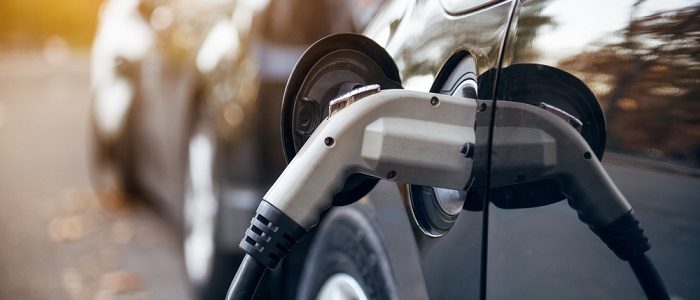
Smart charging for electric vehicles
For a startup in Amsterdam, VORtech developed a system for smart charging of electric vehicles. The aim is to charge as much as possible when the price of electricity is low. VORtech developed both the system and the central algorithm.
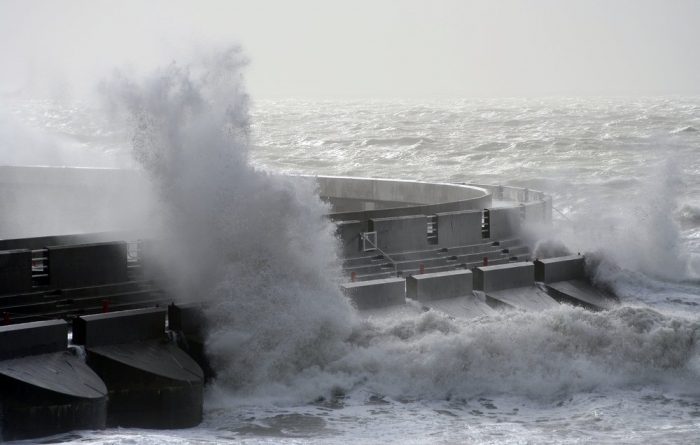
Tidal prediction system
Research institute Deltares has developed a new instrument for tidal prediction for the Dutch Ministry for Infrastructure and the Environment. It is called FEWS-Noordzee and combines a large number of innovations.
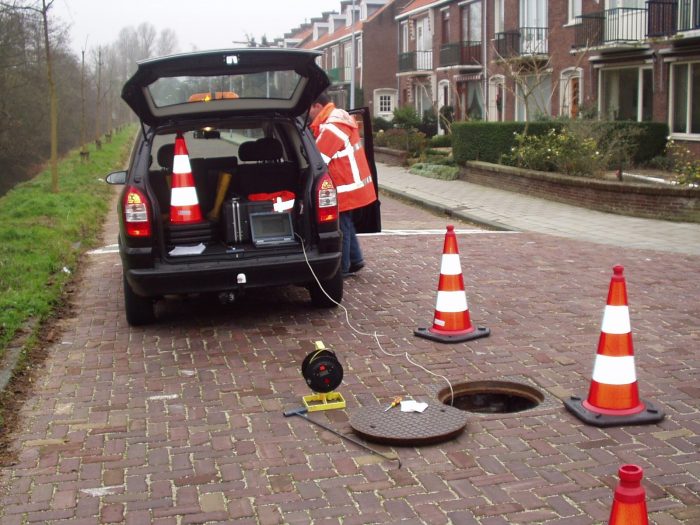
Sewer monitoring
The Dutch sewer system has a length of more than 10.000 km. Monitoring this system is now primarily done by visual inspections. That is expensive and hardly effective. Together with engineering company Witteveen+Bos, VORtech developed methods for automatic monitoring of sewer systems. The methods use observations that are relatively easy to obtain or are even already gathered.
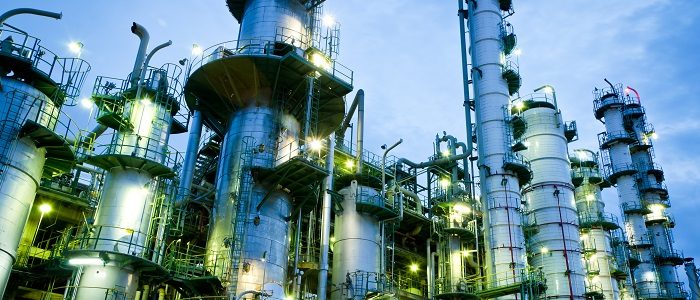
Extending design software for ethylene furnaces
Technips SPYRO® software is a well known package for the design of ethylene furnaces. SPYRO® can also be used in the control of the production process during operation. VORtech supported Technip in extending and speeding up the online version of the software.
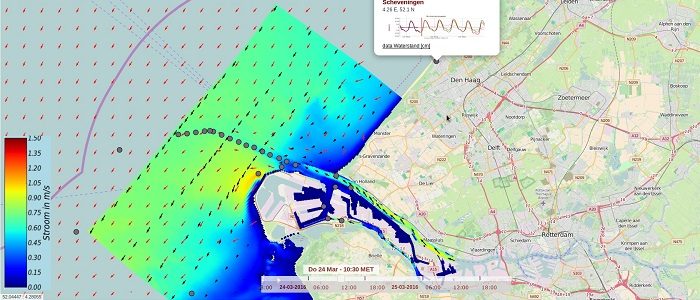
A dashboard for water information
Good information about water levels and flows in coastal areas is important for many stakeholders. VORtech created a new version of the Water Information Viewer (WB-viewer) for this. It gives the Hydro Meteo Centre of the Dutch Rijkswaterstaat an even better dashboard with information on the coastal waters.
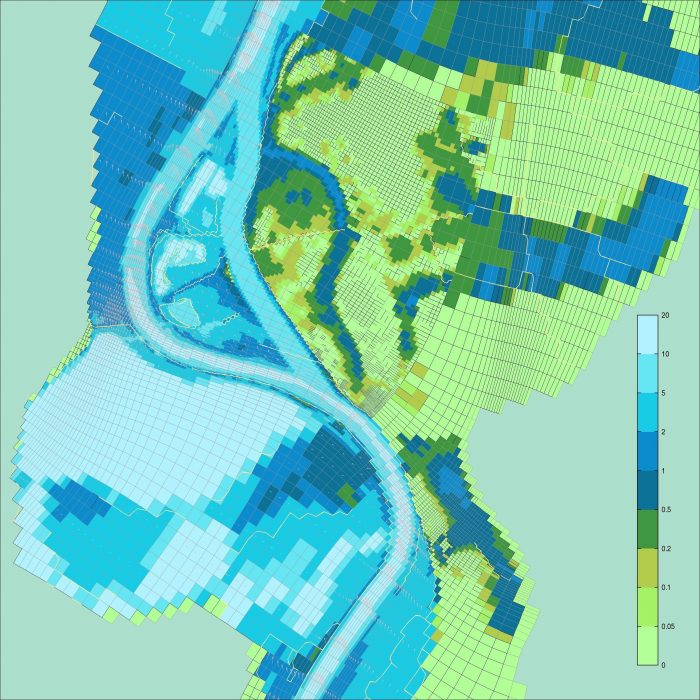
SIMONA maintenance
For over 15 years, VORtech contributed significantly to the the maintenance of the software that the Dutch Ministry of Infrastructure and the Environment uses for its water management.
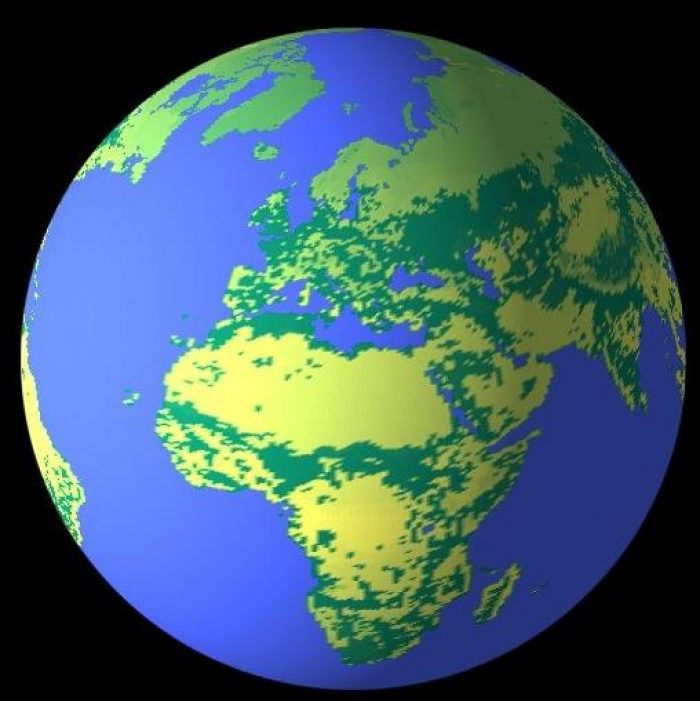
Extending IMAGE
The IMAGE model describes the global environmental changes that take place as a result of human activities. VORtech has made several extensions to the model code.
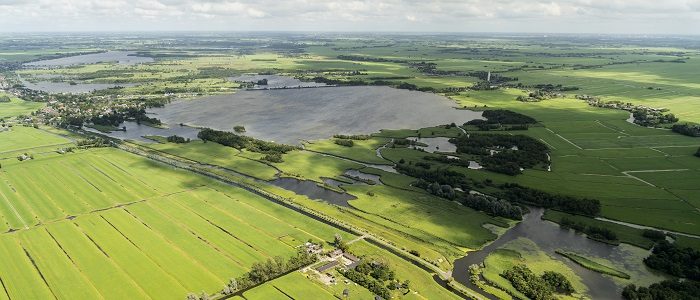
Insights for Water Boards
Water managers in the Netherlands are responsible for an extremely complex water system, where the impact of actions is not always obvious. Therefore water managers need more insight into the relations between variables in their system.
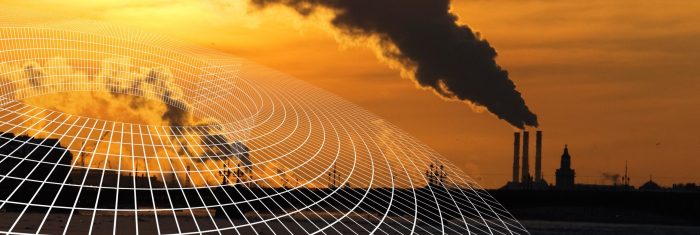
LOTOS-EUROS Optimization
LOTOS-EUROS is a computer model to predict ozone, particulate matter and aerosols for the bottom layer of the atmosphere. It is used for the daily predictions of air quality, among many other applications. The main part of the code was already parallelized. Yet, when asked by KNMI to look into the code, VORtech managed to increase the performance by another 30%.